At first blush, you’d think that Decision Analysts have a lot to contribute to innovation. Innovators face tremendous uncertainty; Decision Analysts are experts at making decisions under uncertain circumstances.
However, Decision Analysts and Innovators look at the world differently. Decision Analysts are deliberative and facilitative: they take time to carefully think through a situation, build models, and recommend one bold move. Many Innovators see that as wasting time speculating rather than actually learning and testing. Agile and Lean approaches direct Innovators to end deliberative processes as quickly as possible in favor of short, iterative learning cycles and rapid adaptation.
A deeper insight synthesizes these worldviews. Many Innovators following the Agile and Lean methods are charging ahead too fast without thinking and getting lost in easily-avoided swamps. Decision Analysis (DA) and its Decision Quality (DQ) framework is a way to create a map of the future: a map of uncertainty and contingency, of where treasures might be buried and where they are not.
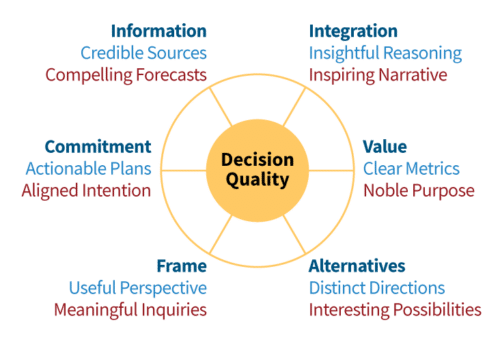
So let’s do a quick review of the Decision Quality (DQ) framework and show how it can apply to innovation. There are six dimensions: Information, Integration, Commitment, Value, Frame, and Alternatives.
Frame
In traditional DA, the frame sets the boundary and perspective on a decision. Poor frames can send groups down ratholes or introduce substantial unintended bias; good ones can generate new opportunity and insight.
Agile movements point out that the frame for Innovation decisions is one of exploration and discovery. The right frame isn’t to make a decision, but rather to open the decision space: this gives Innovators a broad perspective on what major uncertainties they are likely to encounter and how to anticipate them. Then they can design their experiments better and execute more decisively and impactfully.
Alternatives
Many Innovators charge ahead more or less with the first idea they think of. For example, one chemical company I worked with converted every innovative idea into the project “we will invent a new material and sell it”. They were forever making things nobody wanted or getting outmaneuvered by more sophisticated players in the value chain.
It is cognitively easier for teams to “look at their feet” and pick something in front of them to charge at. If that ends up wasting resources on hypotheses that aren’t decisive or useful, Innovators have a way out: they can pivot. It is much better, but harder, to “look at the horizon” and pick a hypothesis based on where you are aiming.
DQ calls for considering several distinct directions and interesting possibilities before making a choice. Exploring broader ideas usually leads to better ones, people with different perspectives can be heard and contribute, and the contrast provides real insight on what really matters in your decisions.
Information
Everyone agrees you need credible sources and good data to support decisions. Still, many Innovators want to make assumptions and then get on with their plans. In innovation, this is a costly mistake.
Decision Quality emphasizes uncertainty in information in its approach. Assumptions essentially cover up uncertainty. Decision Analysis gives you a way to put all the scenarios on the table, and then point your innovation efforts at resolving the uncertainties that demonstrably matter most. This effect shows up in two major ways:
- In the business case. Business cases are littered with assumptions about things like market size, market share, cost structures, etc. By considering ranges of uncertainty and modeling multiple business cases, Innovators can learn which uncertainties matter the most and gain insight into how to drive the upside. They’re often surprised to find they weren’t working on the biggest uncertainties that drive the business case.
- In development. An innovation must get many things right to be successful at all. Of these, only a few are actual proof points (sometimes called kill shots or critical hypotheses). DA defines and assesses these binary success/fail steps productively. DA helps Innovators put the proof points in the right order to uncover failure early and cheaply. That saves Innovators from unintentionally pursuing plans that are likely to fail late and expensively.
Value
In complex decision situations, the question of what you want – your preferences – can be an integral part of problem. Businesses want innovation to drive growth, measured in standard discounted cash flows, revenues or margins. Yet sometimes Innovators get distracted with long lists of for innovation scoring criteria, like size of market, completeness, strategic fit and so on. This approach usually confuses more than it helps (except in the case of early stage idea screening). Most of the “criteria” I usually come across confuse value preferences with inputs for a model. Decision Analysis adds value by simplifying the question of value.
Integration
The various “criteria” that can confuse Innovators are usually inputs to a model. Decision analysis can cut through a lot of this clutter and give real insight into what drives growth, where the risks are, and how to learn most productively.
Decision Analysis has well developed approaches to evaluating multiple alternatives using uncertain information to create recommendations to deliver more of what you value. In an innovation setting, this usually becomes an uncertainty analysis of many business cases. Tools like tornado diagrams, decision trees, option thinking and value of control can be tremendously helpful. The insights from these tools can inspire innovative teams to focus on what matters as they move their projects forward.
Commitment
The final aspect of DQ is commitment. In innovation, commitments are provisional. You rarely go all in. Rather, you invest in experiments and learning. Teams are almost always a bit wrong, but they create new ideas and power up their innovations step by step.
Many organizations are used to a model of “lifecycle funding” in which a project is presumed to continue unless it has a catastrophe. Innovation is more like a continuous series of planned catastrophes, designed to create precisely the conditions of better-informed decisions each time. This framework of stepwise commitment, implicit in a decision tree, is fundamental to innovation and unusual in many organizations.
I call this sort of approach a learning plan. At each stage, the team is going after a terrifying but decisive uncertainty. If they can overcome it or figure out how to drive the upside, then it becomes more and more obvious they should proceed. If the team fails, then it becomes obvious they need to select a different alternative or abandon the project completely.
Because funders know that innovation is uncertain, scary and exciting, proposals that just paint a rosy picture or are hand-wavy about risk don’t inspire confidence. But plans that treat uncertainty and contingency openly and directly build confidence. They show the team is considering multiple directions, has deeply understood the uncertainties it faces, and is truly focusing on the most important things. In short, Decision Quality helps teams find better paths to growth and builds commitment to innovation in an organization.
Innovation is a dangerous and difficult journey with uncertain prospects. It’s dangerous to go alone: take Decision Analysis with you to map your way.